Scientific Publications
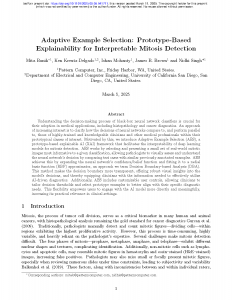
Adaptive Example Selection: Prototype-Based Explainability for Interpretable Mitosis Detection
Understanding the decision-making process of black-box neural network classifiers is crucial for their adoption in medical applications, including histopathology and cancer diagnostics. An approach of increasing interest is to clarify how the decisions of neural networks compare to, and perform parallel to, those of highly-trained and knowledgeable clinicians and other medical professionals within their prototypical classes of interest. Motivated by this, we introduce Adaptive Example Selection (AES), a prototype-based explainable AI (XAI) framework that facilitates the interpretability of deep learning models for mitosis detection. AES works by selecting and presenting a small set of real-world mitotic images most informative to a given classification, allowing pathologists to visually assess and understand the neural network’s decision by comparing test cases with similar previously annotated examples.
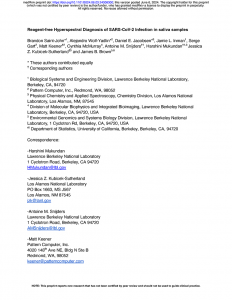
Reagent-free Hyperspectral Diagnosis of SARS-CoV-2 Infection in saliva samples
Rapid, reagent-free pathogen-agnostic diagnostics that can be performed at the point of need are vital for preparedness against future outbreaks. Yet, many current strategies (polymerase chain reaction, lateral flow immunoassays) are pathogen-specific and require reagents; whereas others such as sequencing-based methods; while agnostic, are not (as yet) conducive for use at the point of need. Herein, we present hyperspectral sensing as an opportunity to overcome these barriers, realizing truly agnostic reagent-free diagnostics. This approach can identify both pathogen and host signatures, without complex logistical considerations, in complex clinical samples. The spectral signature of biomolecules across multiple wavelength regimes provides rich biochemical information, which, coupled with machine learning, can facilitate expedited diagnosis of disease states, the feasibility of which is demonstrated here.
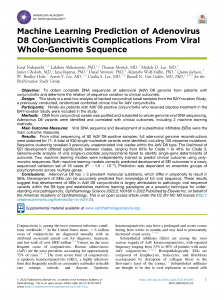
Machine Learning Prediction of Adenovirus D8 Conjunctivitis Complications From Viral Whole-Genome Sequence
To obtain complete DNA sequences of adenoviral (AdV) D8 genome from patients with conjunctivitis and determine the relation of sequence variation to clinical outcomes. This study is a post hoc analysis of banked conjunctival swab samples from the BAYnovation Study, a previously conducted, randomized controlled clinical trial for AdV conjunctivitis. Ninety-six patients with AdV D8-positive conjunctivitis who received placebo treatment in the BAYnovation Study were included in the study. DNA from conjunctival swabs was purified and subjected to whole-genome viral DNA sequencing. Adenovirus D8 variants were identified and correlated with clinical outcomes, including 2 machine learning methods. Viral DNA sequence and development of subepithelial infiltrates (SEIs) were the main outcome measures. From initial sequencing of 80 AdV D8-positive samples, full adenoviral genome reconstructions were obtained for 71. A total of 630 single-nucleotide variants were identified, including 156 missense mutations. Sequence clustering revealed 3 previously unappreciated viral clades within the AdV D8 type. The likelihood of SEI development differed significantly between clades, ranging from 83% for Clade 1 to 46% for Clade 3. Genome-wide analysis of viral single-nucleotide polymorphisms failed to identify single-gene determinants of outcome. Two machine learning models were independently trained to predict clinical outcome using polymorphic sequences. Both machine learning models correctly predicted development of SEI outcomes in a newly sequenced validation set of 16 cases (P = 1.5 X 10-5 ). Prediction was dependent on ensemble groups of polymorphisms across multiple genes. Adenovirus D8 has > or =3 prevalent molecular substrains, which differ in propensity to result in SEIs. Development of SEIs can be accurately predicted from knowledge of full viral sequence. These results suggest that development of SEIs in AdV D8 conjunctivitis is largely attributable to pathologic viral sequence variants within the D8 type and establishes machine learning paradigms as a powerful technique for understanding viral pathogenicity..
Read the paper here.
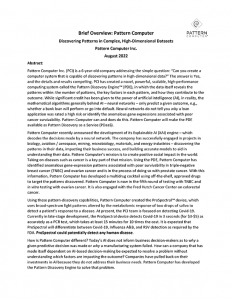
Learning from learning machines: a new generation of AI technology to meet the needs of science
We outline emerging opportunities and challenges to enhance the utility of AI for scientific discovery. The distinct goals of AI for industry versus the goals of AI for science create tension between identifying patterns in data versus discovering patterns in the world from data. If we address the fundamental challenges associated with “bridging the gap” between domain-driven scientific models and data-driven AI learning machines, then we expect that these AI models can transform hypothesis generation, scientific discovery, and the scientific process itself.
Read the paper here.